Table of Contents
As Artificial Intelligence (AI) becomes indispensable across various industries, concerns over its ecological impact are growing. From energy consumption to electronic waste, the environmental footprint of AI can vary greatly depending on the deployment model. In this article, we’ll examine how different AI models impact the environment and explore which options are more sustainable.
What are the four AI usage models?
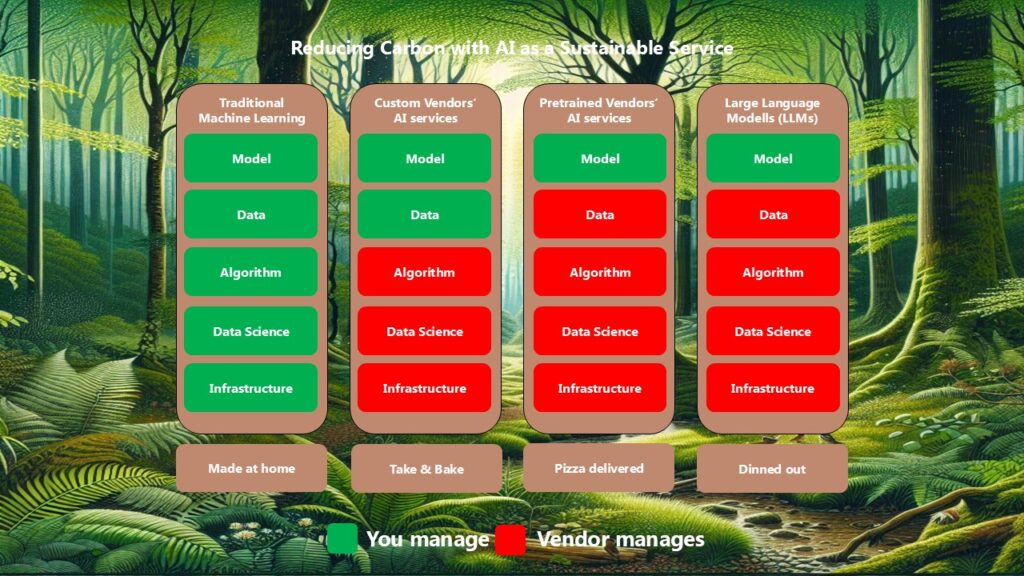
The “Reducing Carbon with AI as a Sustainable Service” graphic compares four distinct AI deployment models: Traditional Machine Learning, Custom Vendors’ AI Services, Pretrained Vendors’ AI Services, and Large Language Models (LLMs). These models are illustrated using a “pizza model” analogy, which reflects the levels of responsibility that a company or provider manages:
- Traditional Machine Learning (“Made at home”): The user is responsible for the entire AI stack – model, data, algorithm, data science, and infrastructure.
- Custom Vendors’ AI Services (“Take & Bake”): The provider supplies certain components, while the user retains control over the model and data.
- Pretrained Vendors’ AI Services (“Pizza delivered”): The vendor manages almost everything, except for the model, which the user can customize.
- Large Language Models (LLMs, “Dined out”): The provider manages all components, leaving the user to simply leverage the ready-to-use model.
Each model has distinct ecological impacts, with vendor-managed models often being more sustainable due to shared, optimized resources.
Key environmental factors and carbon footprint of AI models
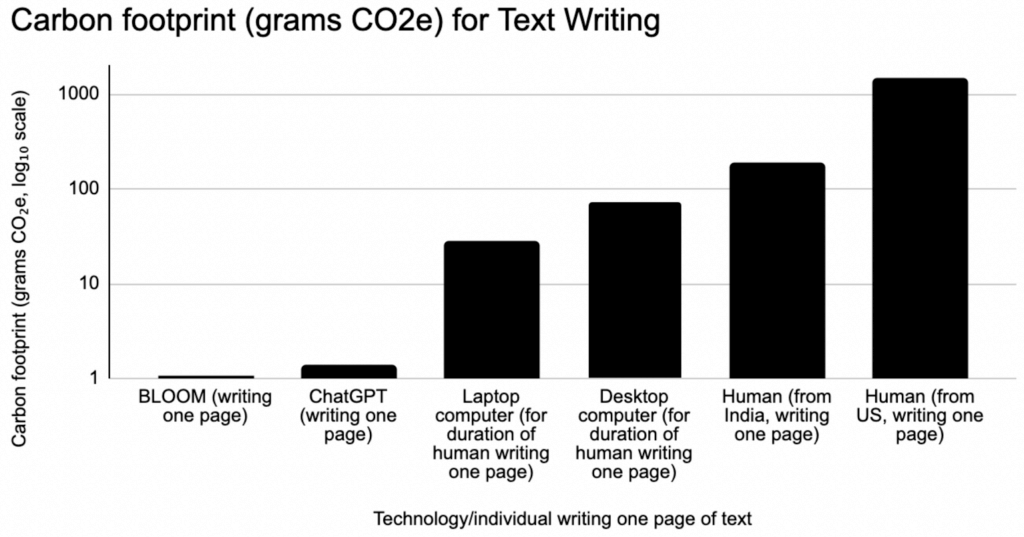
Energy consumption and CO₂ emissions
Each AI component (model, data, algorithms, data science, and infrastructure) requires energy, directly impacting the CO₂ footprint. In Traditional Machine Learning, where users manage everything locally, energy consumption can be high, as this setup often lacks the energy efficiency found in cloud solutions. Locally managed infrastructures can result in a significant carbon footprint due to the less efficient hardware and cooling systems.
In contrast, Large Language Models typically use the provider’s optimized data centers, which are often energy-efficient or even carbon-neutral. Providers frequently invest in renewable energy and advanced cooling technologies to reduce emissions, making this model more ecologically sustainable.
Data centre utilisation and efficiency
Cloud-based AI solutions and providers with specialized infrastructures often have lower CO₂ footprints. Companies like Google, Microsoft, and Amazon have invested in energy-efficient data centers that leverage ambient temperature cooling and advanced hardware designed specifically for machine learning.
With Traditional Machine Learning, companies often require their own infrastructure, which is both resource-intensive and less efficient. Building and maintaining machine learning-specific infrastructure is challenging and can significantly increase the overall CO₂ footprint.
Material consumption and electronic waste
Local hardware for AI often needs replacement every few years to keep up with new algorithms, leading to increased electronic waste. This is particularly an issue in Traditional Machine Learning and Custom Vendors’ AI Services. In centralized, cloud-based systems, large providers have efficient hardware management and replacement cycles, reducing the overall material consumption and electronic waste.
Data storage and processing
The data storage and processing demands of AI increase with data volume. In Traditional Machine Learning and Custom Vendors’ AI Services, users typically need local storage, which can drive up energy consumption and CO₂ emissions. Cloud models such as Pretrained Vendors’ AI Services and LLMs utilize shared, scalable infrastructure that is often optimized for energy efficiency, resulting in a lower environmental impact.
Cooling and environmental impact
Cooling is a major energy consumer for AI applications. LLMs and other cloud solutions often use modern, energy-efficient cooling systems. In contrast, local models lack this technology, relying instead on traditional cooling, which can significantly raise the ecological footprint.
Towards a sustainable AI future
Choosing the right AI deployment model can make a significant difference for the environment. Companies should consider not only performance but also the ecological footprint of their AI systems. For those prioritizing sustainability, LLMs and Pretrained Vendors’ AI Services may be the optimal choice. Benefits of these models include:
- Improved Energy Efficiency: Leveraging highly optimized, often CO₂-neutral data centers.
- Reduced Material Waste: Lower hardware demand and longer replacement cycles.
- Less Electronic Waste: Centralized systems generally require less frequent hardware replacements.
To further reduce ecological impact, companies should select climate-neutral providers that prioritize renewable energy. Thoughtful AI deployment can significantly reduce the environmental footprint.
Environmental impact of machine learning models
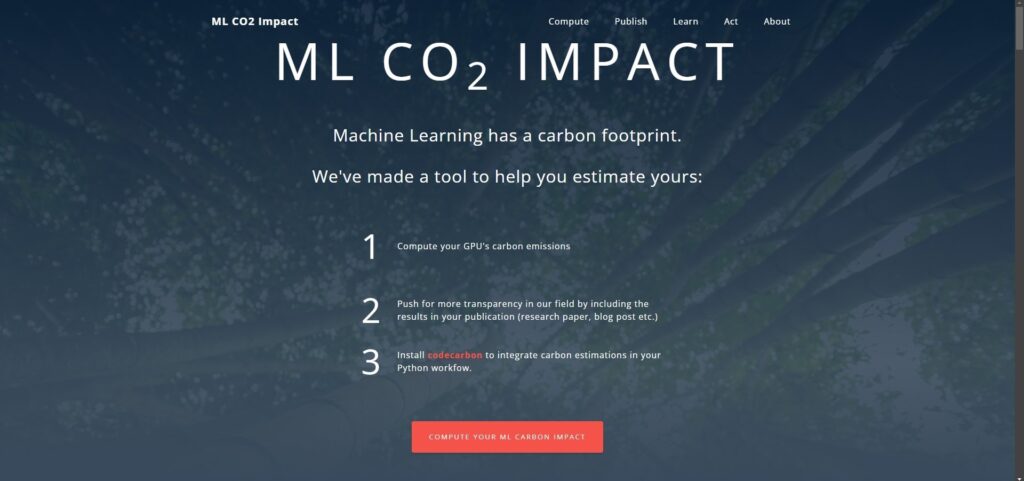
Use the Machine Learning Impact Calculator to easily estimate the carbon footprint of your work. By inputting details like cloud provider, region, and hardware, you can see the environmental cost of your training process and make informed, eco-friendly choices. Take a step toward sustainable AI today—calculate your model’s carbon footprint now!
Conclusion
The sustainability of AI systems largely depends on the chosen deployment model. Models where providers handle infrastructure and data storage tend to be more sustainable than locally operated ones. Transitioning to centralized, cloud-based AI models can reduce CO₂ emissions and other environmental impacts in the long term. Ultimately, companies must consider ecological factors when choosing their AI approach to contribute to a more sustainable future.
Literature
- BBC – AI drives 48% increase in Google emissions: https://www.bbc.com/news/articles/c51yvz51k2xo
- E-waste challenges of generative artificial intelligence: https://www.nature.com/articles/s43588-024-00712-6
- Albert Barron’s pizza as a service: https://www.linkedin.com/pulse/20140730172610-9679881-pizza-as-a-service/
- Dainalytix research group: AI as a Service: Serving Intelligence by the Slice of Pizza: https://www.dainalytix.com/blog/ai-as-a-service-serving-intelligence-by-the-slice-of-pizza/
- AI’s carbon footprint is bigger than you think – https://www.technologyreview.com/2023/12/05/1084417/ais-carbon-footprint-is-bigger-than-you-think/
- Machine Learning Impact Calculator: https://mlco2.github.io/impact/#home
- The carbon emissions of writing and illustrating are lower for AI than for humans: https://www.nature.com/articles/s41598-024-54271-x
- Toward artificial intelligence and machine learning-enabled frameworks for improved predictions of lifecycle environmental impacts of functional materials and devices: https://link.springer.com/article/10.1557/s43579-023-00480-w